Artificial intelligence is now being used to make decisions about lives, livelihoods, and interactions in the real world in ways that pose real risks to people.
We were all skeptics once. Not that long ago, conventional wisdom held that machine intelligence showed great promise, but it was always just a few years away. Today there is absolute faith that the future has arrived.
It’s not that surprising with cars that (sometimes and under certain conditions) drive themselves and software that beats humans at games like chess and Go. You can’t blame people for being impressed.
But board games, even complicated ones, are a far cry from the messiness and uncertainty of real-life, and autonomous cars still aren’t actually sharing the road with us (at least not without some catastrophic failures).
AI is being used in a surprising number of applications, making judgments about job performance, hiring, loans, and criminal justice among many others. Most people are not aware of the potential risks in these judgments. They should be. There is a general feeling that technology is inherently neutral — even among many of those developing AI solutions. But AI developers make decisions and choose tradeoffs that affect outcomes. Developers are embedding ethical choices within the technology but without thinking about their decisions in those terms.
These tradeoffs are usually technical and subtle, and the downstream implications are not always obvious at the point the decisions are made.
The fatal Uber accident in Tempe, Arizona, is a (not-subtle) but good illustrative example that makes it easy to see how it happens.
The autonomous vehicle system actually detected the pedestrian in time to stop but the developers had tweaked the emergency braking system in favor of not braking too much, balancing a tradeoff between jerky driving and safety. The Uber developers opted for the more commercially viable choice. Eventually autonomous driving technology will improve to a point that allows for both safety and smooth driving, but will we put autonomous cars on the road before that happens? Profit interests are pushing hard to get them on the road immediately.
Physical risks pose an obvious danger, but there has been real harm from automated decision-making systems as well. AI does, in fact, have the potential to benefit the world. Ideally, we mitigate for the downsides in order to get the benefits with minimal harm.
A significant risk is that we advance the use of AI technology at the cost of reducing individual human rights. We’re already seeing that happen. One important example is that the right to appeal judicial decisions is weakened when AI tools are involved. In many other cases, individuals don’t even know that a choice not to hire, promote, or extend a loan to them was informed by a statistical algorithm.
Buyer Beware
Buyers of the technology are at a disadvantage when they know so much less about it than the sellers do. For the most part decision makers are not equipped to evaluate intelligent systems. In economic terms, there is an information asymmetry that puts AI developers in a more powerful position over those who might use it. (Side note: the subjects of AI decisions generally have no power at all.) The nature of AI is that you simply trust (or not) the decisions it makes. You can’t ask technology why it decided something or if it considered other alternatives or suggest hypotheticals to explore variations on the question you asked. Given the current trust in technology, vendors’ promises about a cheaper and faster way to get the job done can be very enticing.
So far, we as a society have not had a way to assess the value of algorithms against the costs they impose on society. There has been very little public discussion even when government entities decide to adopt new AI solutions. Worse than that, information about the data used for training the system plus its weighting schemes, model selection, and other choices vendors make while developing the software are deemed trade secrets and therefore not available for discussion.
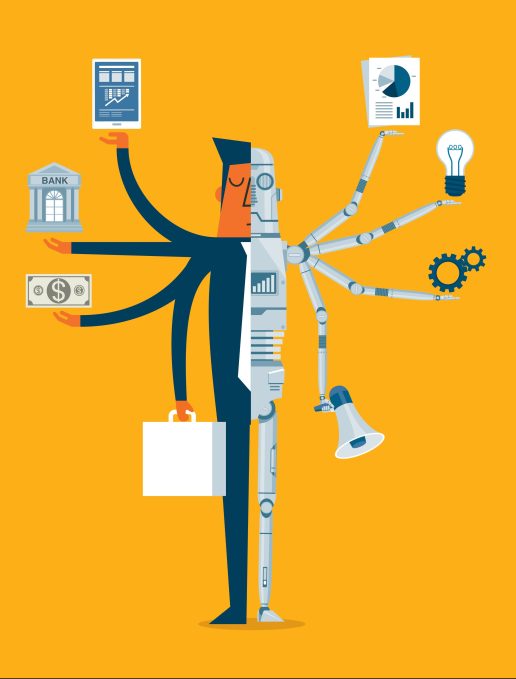
Image via Getty Images / sorbetto
The Yale Journal of Law and Technology published a paper by Robert Brauneis and Ellen P. Goodman where they describe their efforts to test the transparency around government adoption of data analytics tools for predictive algorithms. They filed forty-two open records requests to various public agencies about their use of decision-making support tools.
Their “specific goal was to assess whether open records processes would enable citizens to discover what policy judgments these algorithms embody and to evaluate their utility and fairness”. Nearly all of the agencies involved were either unwilling or unable to provide information that could lead to an understanding of how the algorithms worked to decide citizens’ fates. Government record-keeping was one of the biggest problems, but companies’ aggressive trade secret and confidentiality claims were also a significant factor.
Using data-driven risk assessment tools can be useful especially in cases identifying low-risk individuals who can benefit from reduced prison sentences. Reduced or waived sentences alleviate stresses on the prison system and benefit the individuals, their families, and communities as well. Despite the possible upsides, if these tools interfere with Constitutional rights to due process, they are not worth the risk.
All of us have the right to question the accuracy and relevance of information used in judicial proceedings and in many other situations as well. Unfortunately for the citizens of Wisconsin, the argument that a company’s profit interest outweighs a defendant’s right to due process was affirmed by that state’s supreme court in 2016.
Fairness is in the Eye of the Beholder
Of course, human judgment is biased too. Indeed, professional cultures have had to evolve to address it. Judges for example, strive to separate their prejudices from their judgments, and there are processes to challenge the fairness of judicial decisions.
In the United States, the 1968 Fair Housing Act was passed to ensure that real-estate professionals conduct their business without discriminating against clients. Technology companies do not have such a culture. Recent news has shown just the opposite. For individual AI developers, the focus is on getting the algorithms correct with high accuracy for whatever definition of accuracy they assume in their modeling.
I recently listened to a podcast where the conversation wondered whether talk about bias in AI wasn’t holding machines to a different standard than humans—seeming to suggest that machines were being put at a disadvantage in some imagined competition with humans.
As true technology believers, the host and guest eventually concluded that once AI researchers have solved the machine bias problem, we’ll have a new, even better standard for humans to live up to, and at that point the machines can teach humans how to avoid bias. The implication is that there is an objective answer out there, and while we humans have struggled to find it, the machines can show us the way. The truth is that in many cases there are contradictory notions about what it means to be fair.
A handful of research papers have come out in the past couple of years that tackle the question of fairness from a statistical and mathematical point-of-view. One of the papers, for example, formalizes some basic criteria to determine if a decision is fair.
In their formalization, in most situations, differing ideas about what it means to be fair are not just different but actually incompatible. A single objective solution that can be called fair simply doesn’t exist, making it impossible for statistically trained machines to answer these questions. Considered in this light, a conversation about machines giving human beings lessons in fairness sounds more like theater of the absurd than a purported thoughtful conversation about the issues involved.
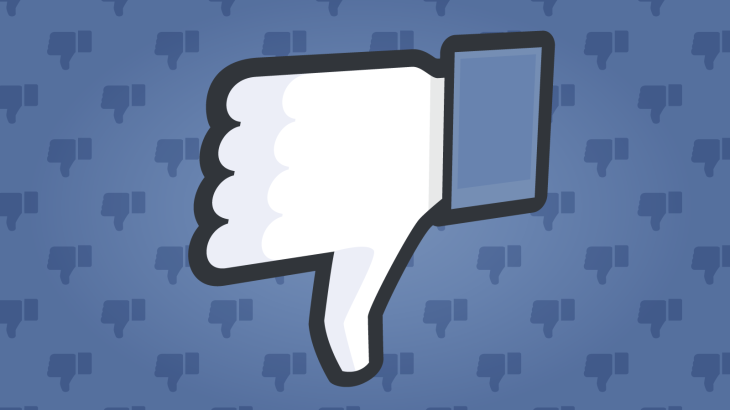
Image courtesy of TechCrunch/Bryce Durbin
When there are questions of bias, a discussion is necessary. What it means to be fair in contexts like criminal sentencing, granting loans, job and college opportunities, for example, have not been settled and unfortunately contain political elements. We’re being asked to join in an illusion that artificial intelligence can somehow de-politicize these issues. The fact is, the technology embodies a particular stance, but we don’t know what it is.
Technologists with their heads down focused on algorithms are determining important structural issues and making policy choices. This removes the collective conversation and cuts off input from other points-of-view. Sociologists, historians, political scientists, and above all stakeholders within the community would have a lot to contribute to the debate. Applying AI for these tricky problems paints a veneer of science that tries to dole out apolitical solutions to difficult questions.
Who Will Watch the (AI) Watchers?
One major driver of the current trend to adopt AI solutions is that the negative externalities from the use of AI are not borne by the companies developing it. Typically, we address this situation with government regulation. Industrial pollution, for example, is restricted because it creates a future cost to society. We also use regulation to protect individuals in situations where they may come to harm.
Both of these potential negative consequences exist in our current uses of AI. For self-driving cars, there are already regulatory bodies involved, so we can expect a public dialog about when and in what ways AI driven vehicles can be used. What about the other uses of AI? Currently, except for some action by New York City, there is exactly zero regulation around the use of AI. The most basic assurances of algorithmic accountability are not guaranteed for either users of technology or the subjects of automated decision making.
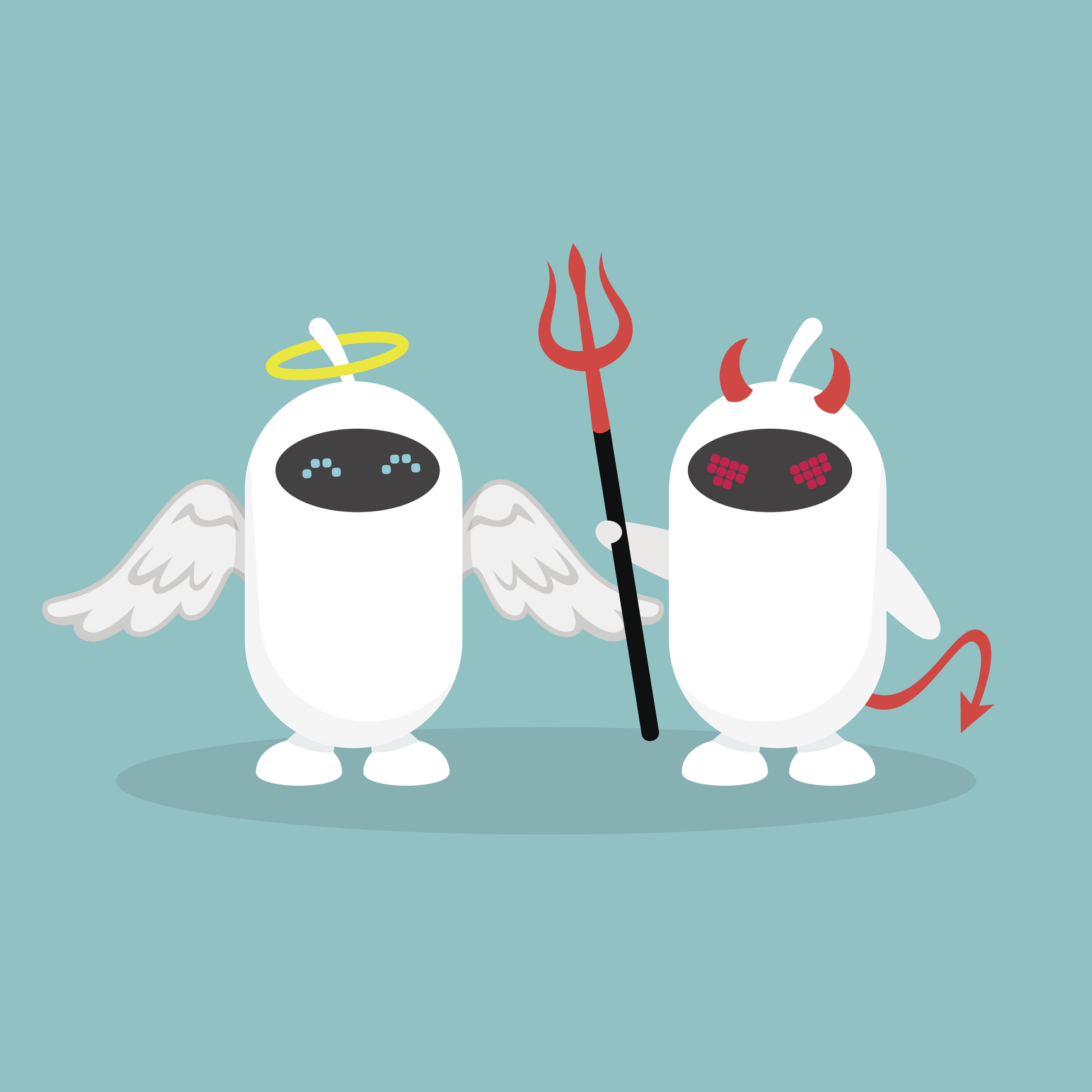
Image via Getty Images / nadia_bormotova
Unfortunately, we can’t leave it to companies to police themselves. Facebook’s slogan, “Move fast and break things” has been retired, but the mindset and the culture persist throughout Silicon Valley. An attitude of doing what you think is best and apologizing later continues to dominate.
This has apparently been effective when building systems to upsell consumers or connect riders with drivers. It becomes completely unacceptable when you make decisions affecting people’s lives. Even if well-intentioned, the researchers and developers writing the code don’t have the training or, at the risk of offending some wonderful colleagues, the inclination to think about these issues.
I’ve seen firsthand too many researchers who demonstrate a surprising nonchalance about the human impact. I recently attended an innovation conference just outside of Silicon Valley. One of the presentations included a doctored video of a very famous person delivering a speech that never actually took place. The manipulation of the video was completely imperceptible.
When the researcher was asked about the implications of deceptive technology, she was dismissive of the question. Her answer was essentially, “I make the technology and then leave those questions to the social scientists to work out.” This is just one of the worst examples I’ve seen from many researchers who don’t have these issues on their radars. I suppose that requiring computer scientists to double major in moral philosophy isn’t practical, but the lack of concern is striking.
Recently we learned that Amazon abandoned an in-house technology that they had been testing to select the best resumes from among their applicants. Amazon discovered that the system they created developed a preference for male candidates, in effect, penalizing women who applied. In this case, Amazon was sufficiently motivated to ensure their own technology was working as effectively as possible, but will other companies be as vigilant?
As a matter of fact, Reuters reports that other companies are blithely moving ahead with AI for hiring. A third-party vendor selling such technology actually has no incentive to test that it’s not biased unless customers demand it, and as I mentioned, decision makers are mostly not in a position to have that conversation. Again, human bias plays a part in hiring too. But companies can and should deal with that.
With machine learning, they can’t be sure what discriminatory features the system might learn. Absent the market forces, unless companies are compelled to be transparent about the development and their use of opaque technology in domains where fairness matters, it’s not going to happen.
Accountability and transparency are paramount to safely using AI in real-world applications. Regulations could require access to basic information about the technology. Since no solution is completely accurate, the regulation should allow adopters to understand the effects of errors. Are errors relatively minor or major? Uber’s use of AI killed a pedestrian. How bad is the worst-case scenario in other applications? How are algorithms trained? What data was used for training and how was it assessed to determine its fitness for the intended purpose? Does it truly represent the people under consideration? Does it contain biases? Only by having access to this kind of information can stakeholders make informed decisions about appropriate risks and tradeoffs.
At this point, we might have to face the fact that our current uses of AI are getting ahead of its capabilities and that using it safely requires a lot more thought than it’s getting now.
from TechCrunch https://ift.tt/2U20EPb
via IFTTT
No comments:
Post a Comment